Deep Himmelblau
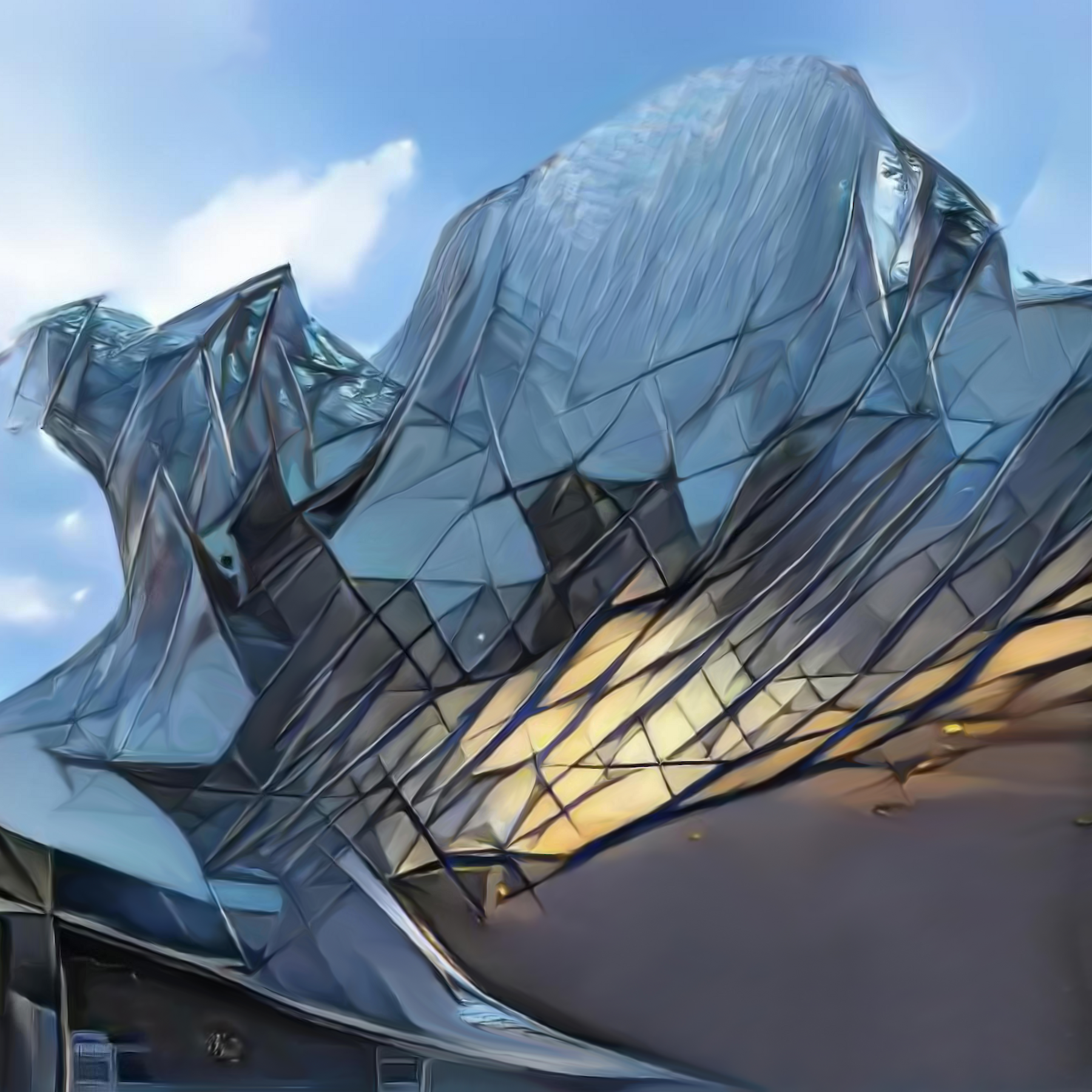
While developments in artificial intelligence (AI) mean computers can be trained on certain creativity criteria, the degree to which AI can develop its own sense of creativity it’s still something to enquire about. Can AI be taught without guidance how to create?
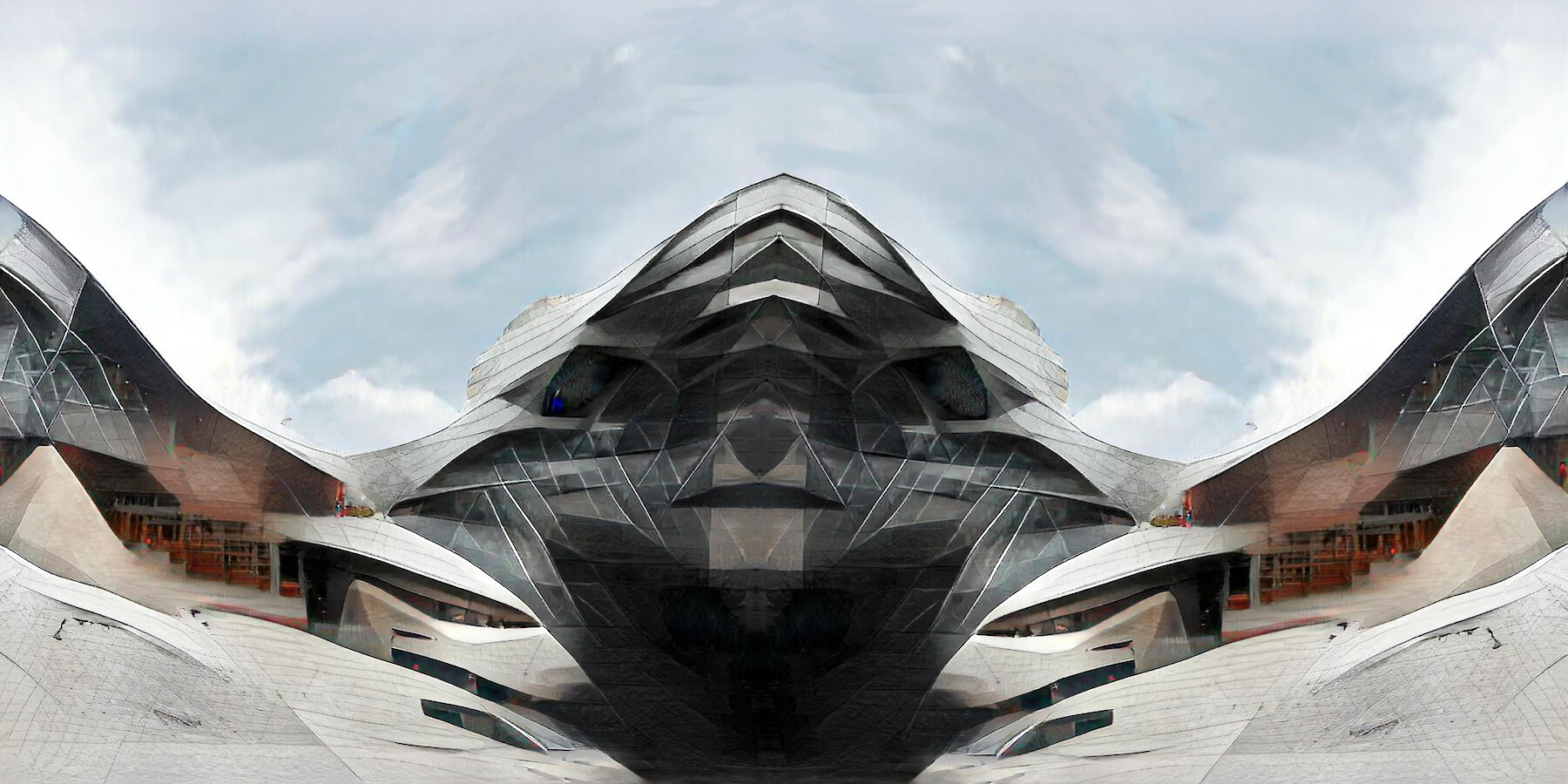
Cloud augmentation of Deep Himmelblau universe
Coop Himmelb(l)au
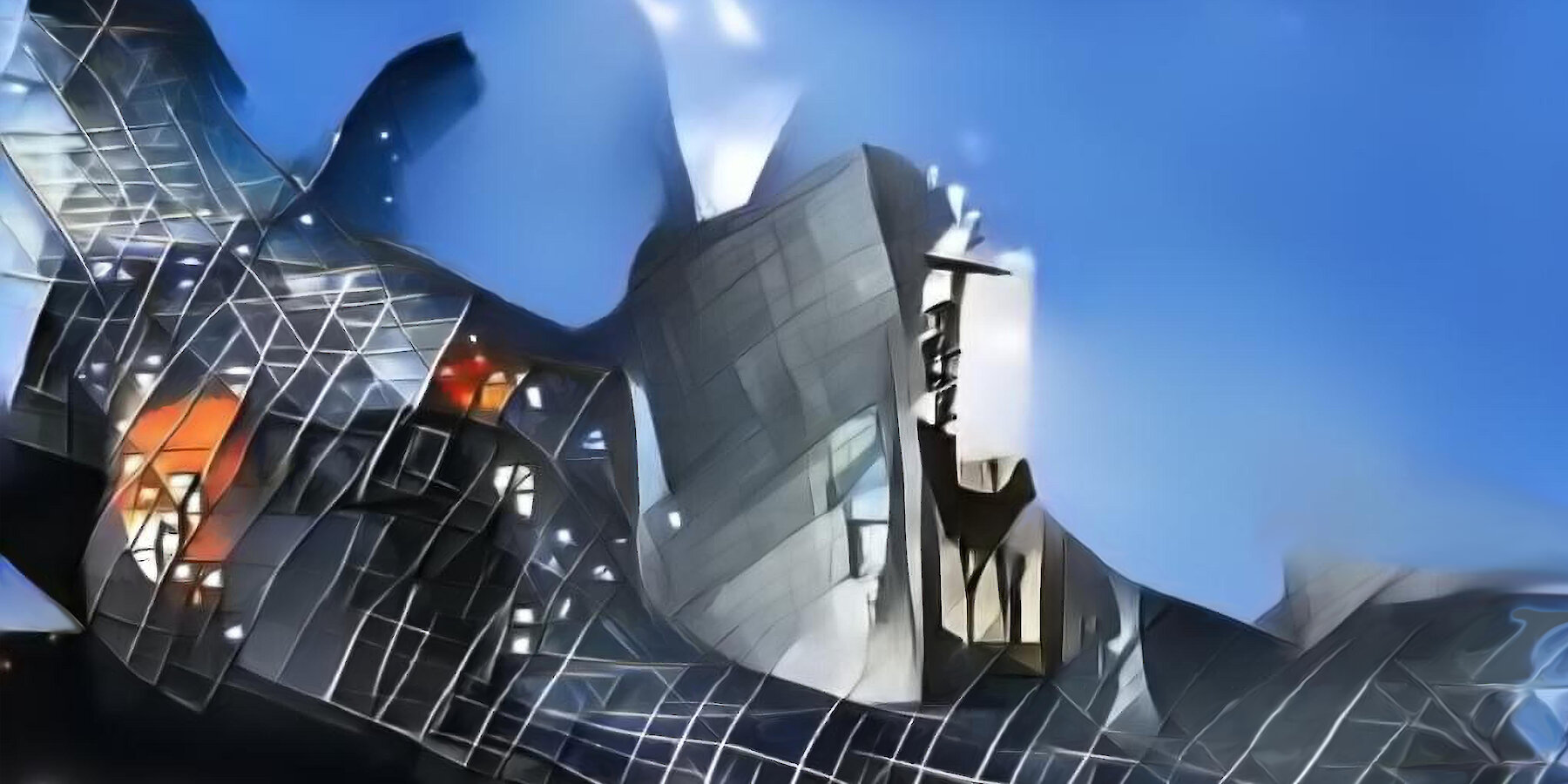
引言
Much like feathers were developed by nature without dinosaurs ever thinking about flying, AI - which I like to call "Architectural Intelligence" - is a tool that will one day allow us, architects, to fly.
While developments in AI mean computers can be trained on certain creativity criteria, the degree to which AI can develop its own sense of creativity it’s still something to enquire about. Can AI be taught without guidance how to create? Can AI be taught how to interpret things? Can AI be taught how to reinterpret representations from one domain to another, similar to how architects are inspired by concepts outside their architectural domain? Teaching computers to be creative is inherently different from how people create, but we do not yet know much about our own creative methodology.
Our perceptions and our conscious visual representations of the reality are not a direct mapping of the real world. Humans interpret reality through reconstructions and interpretations based on past experiences. Our past experiences act as a frame / filter on our way of interpreting, understanding and perceiving the real world. Our training as architects operates as a filter / frame in the way we perceive the world, the way we interpret it and the way we draw inspiration from it.
One very common practice in design and architecture is that a designer learns, consciously or unconsciously semantic representation of one domain, reinterpret that representation through a particular filter e.g. architectural style, architectural culture etc, and translate it to a different domain.
While humans unconsciously are capable to recognize and disentangle various semantic features of what they perceive, neural networks are capable of having similar behavior after learning from a large enough set of samples. Some Networks learn automatically to separate/disentangle various semantic features of a dataset and afterwards enable specific features to be separated and managed on a particular level. In addition, machines exposed to large sample sets can discover perceptual deficiencies in human recognition capabilities. Can this innate capacity augment the creativity and interpretation of the designer?
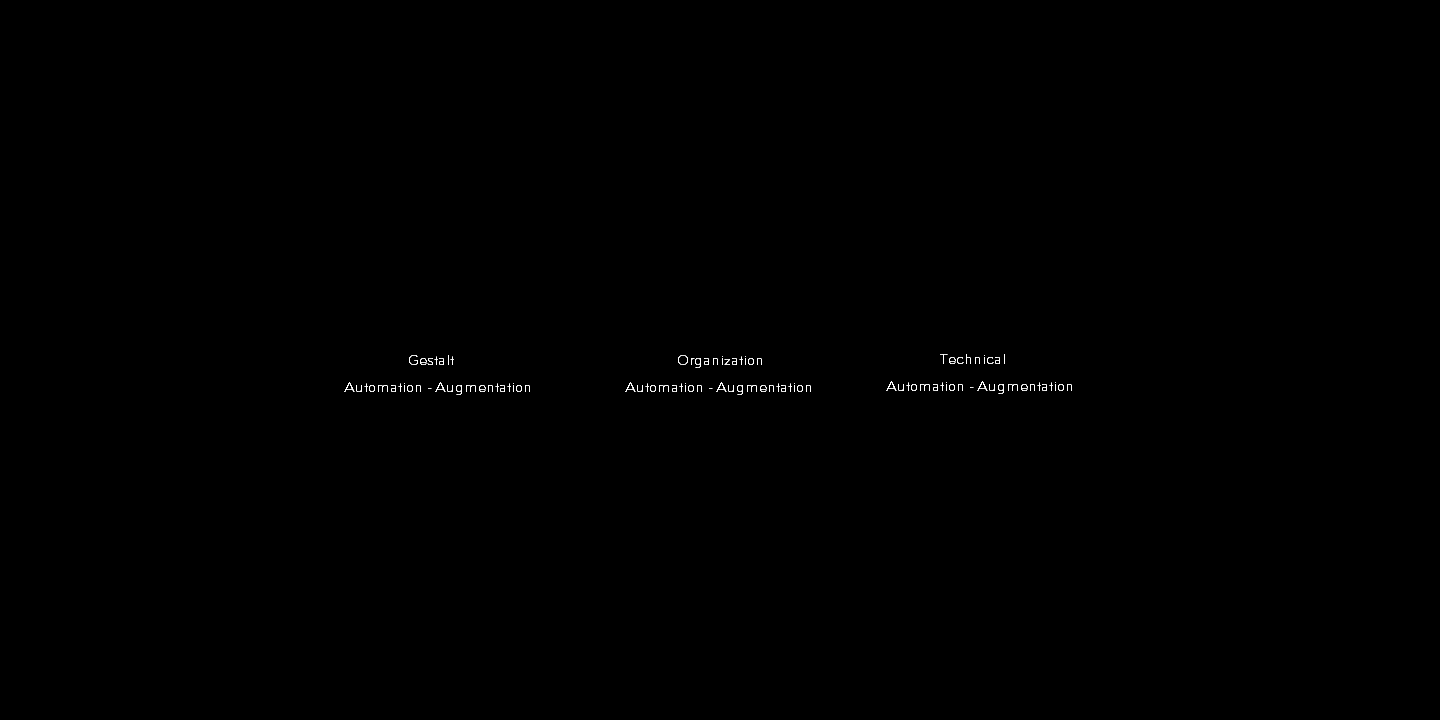
Representation learning on the field of various 3D geometries
Coop Himmelb(l)au
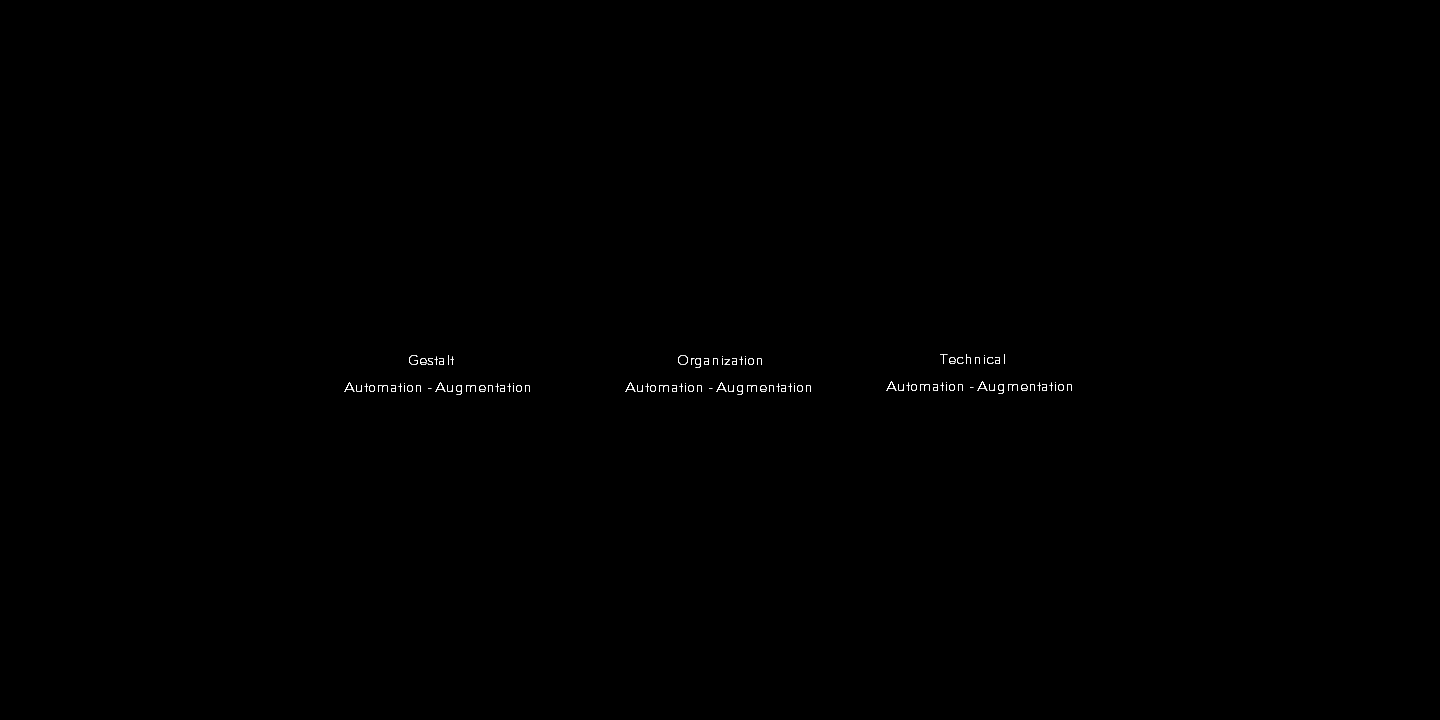
Representation learning on single 3D form - zoom in
Coop Himmelb(l)au
Deep Himmelblau Universe
成员
沃尔夫 狄 普瑞克斯
联合创建人、首席执行官、主持设计师
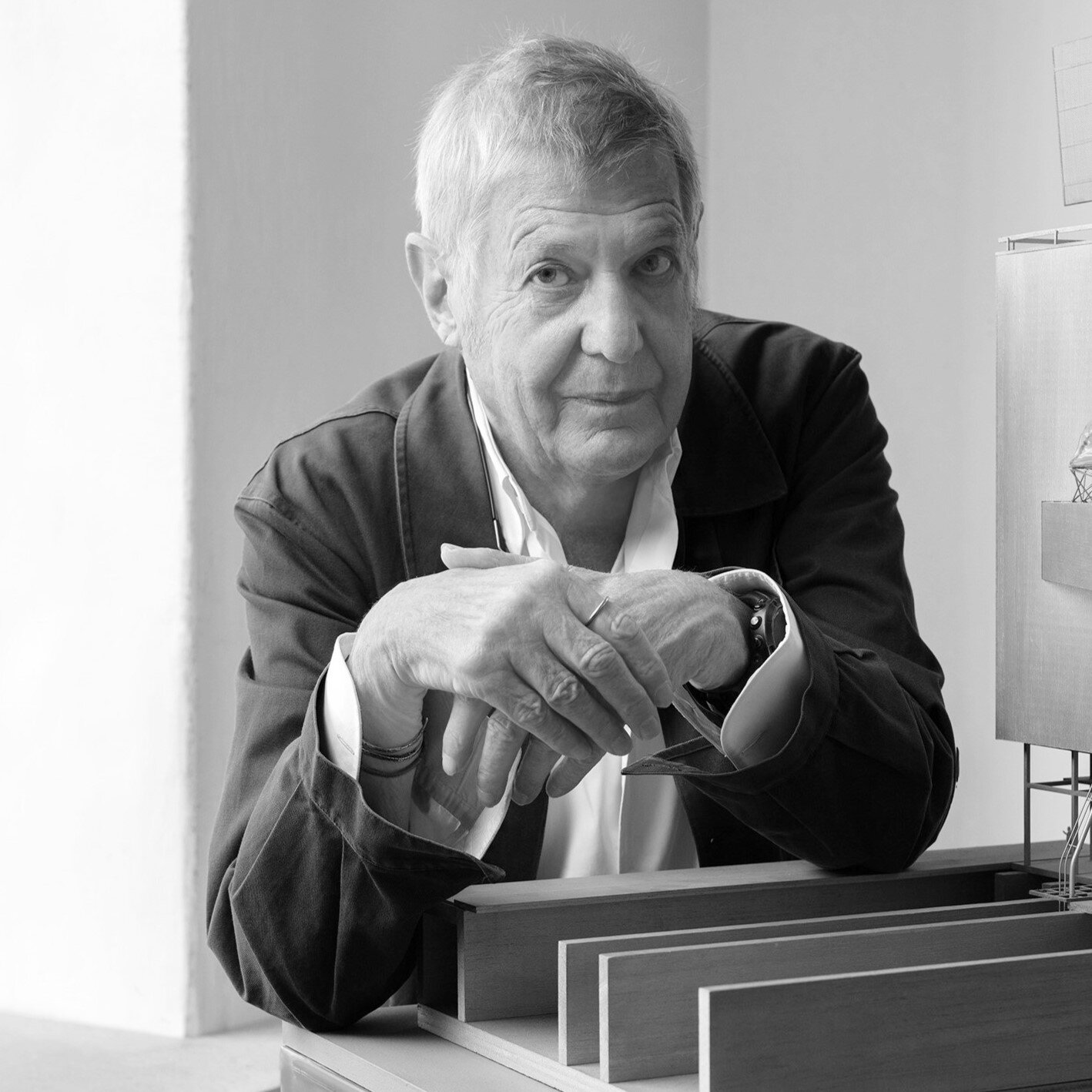
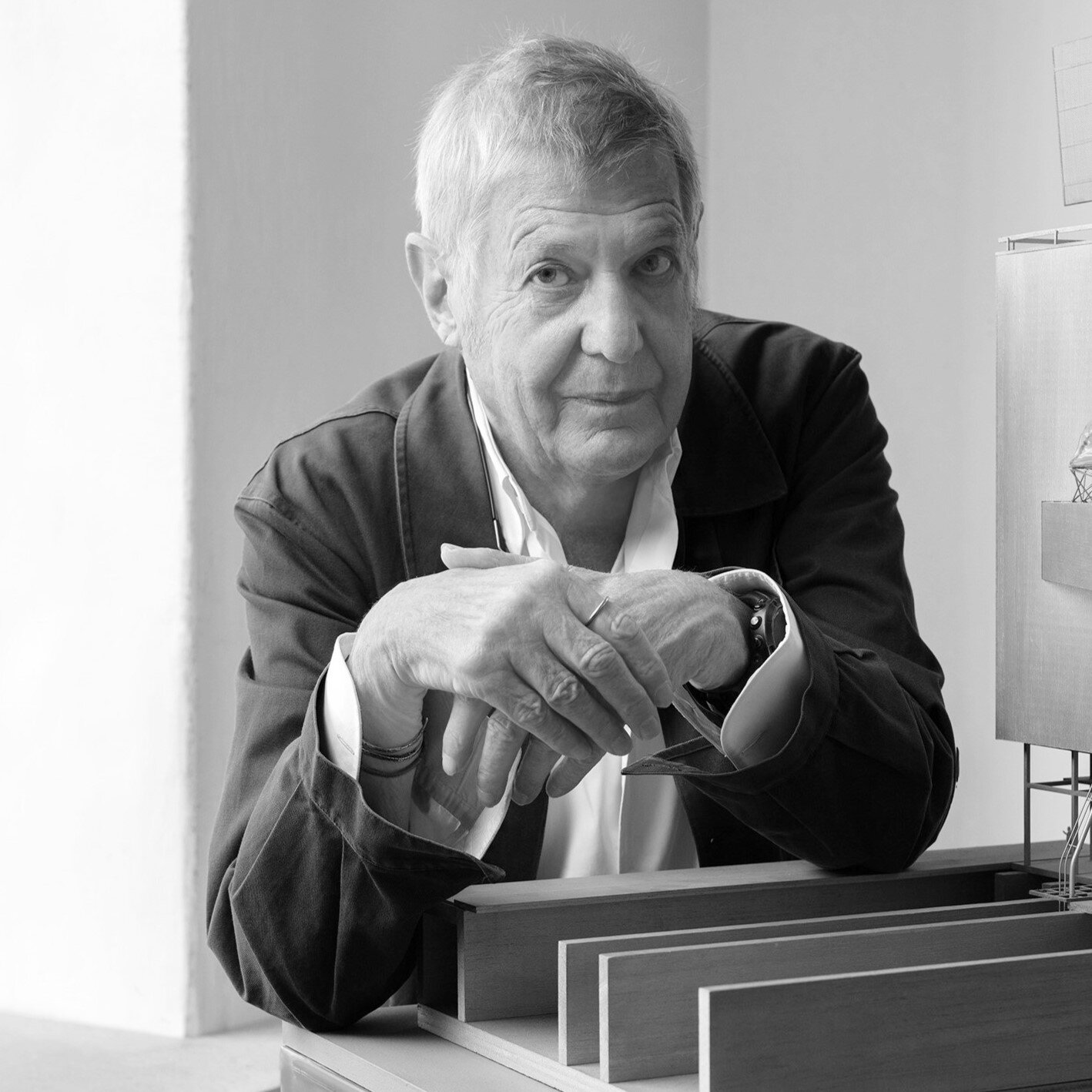
卡罗琳 施密德鲍尓
设计合伙人、研究总监
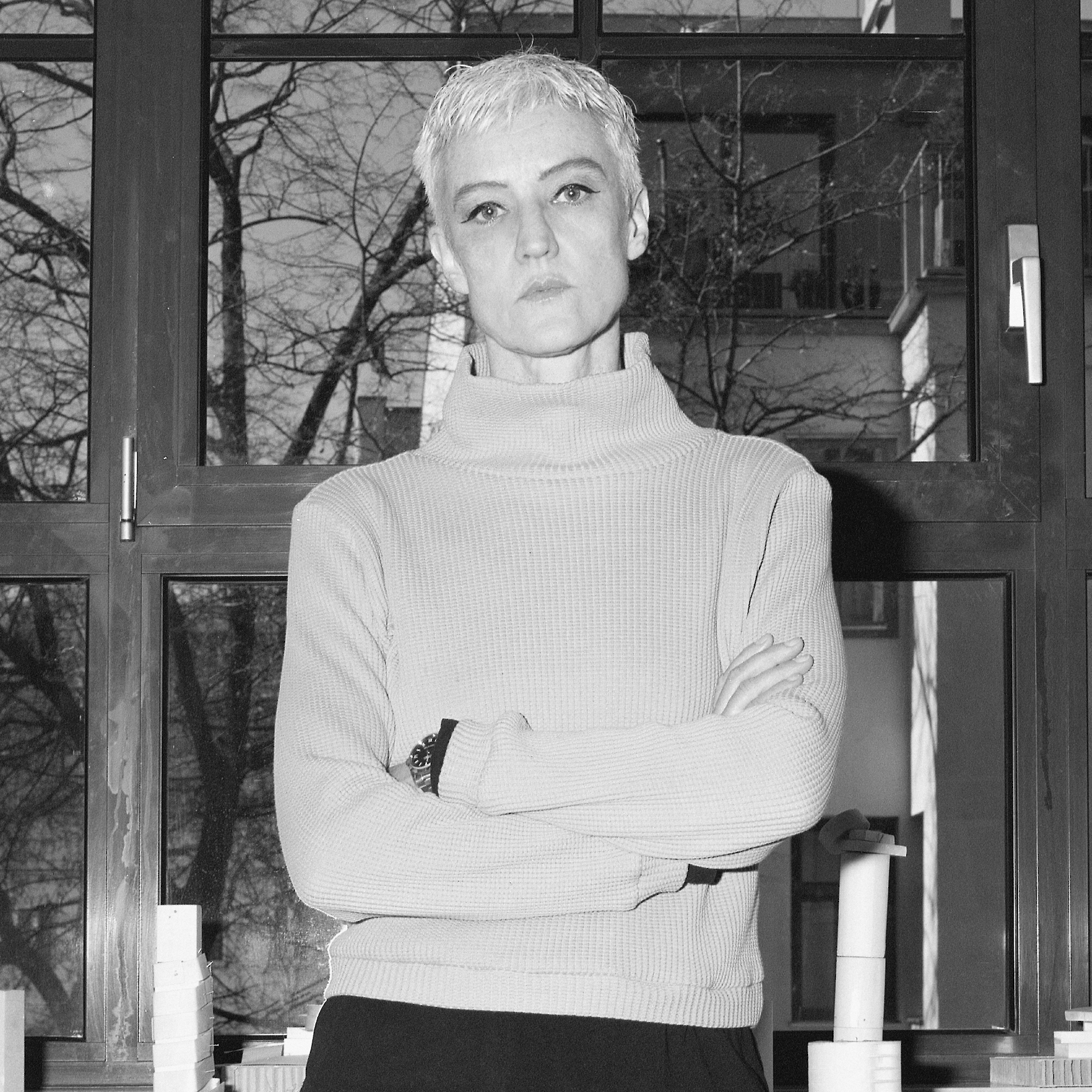
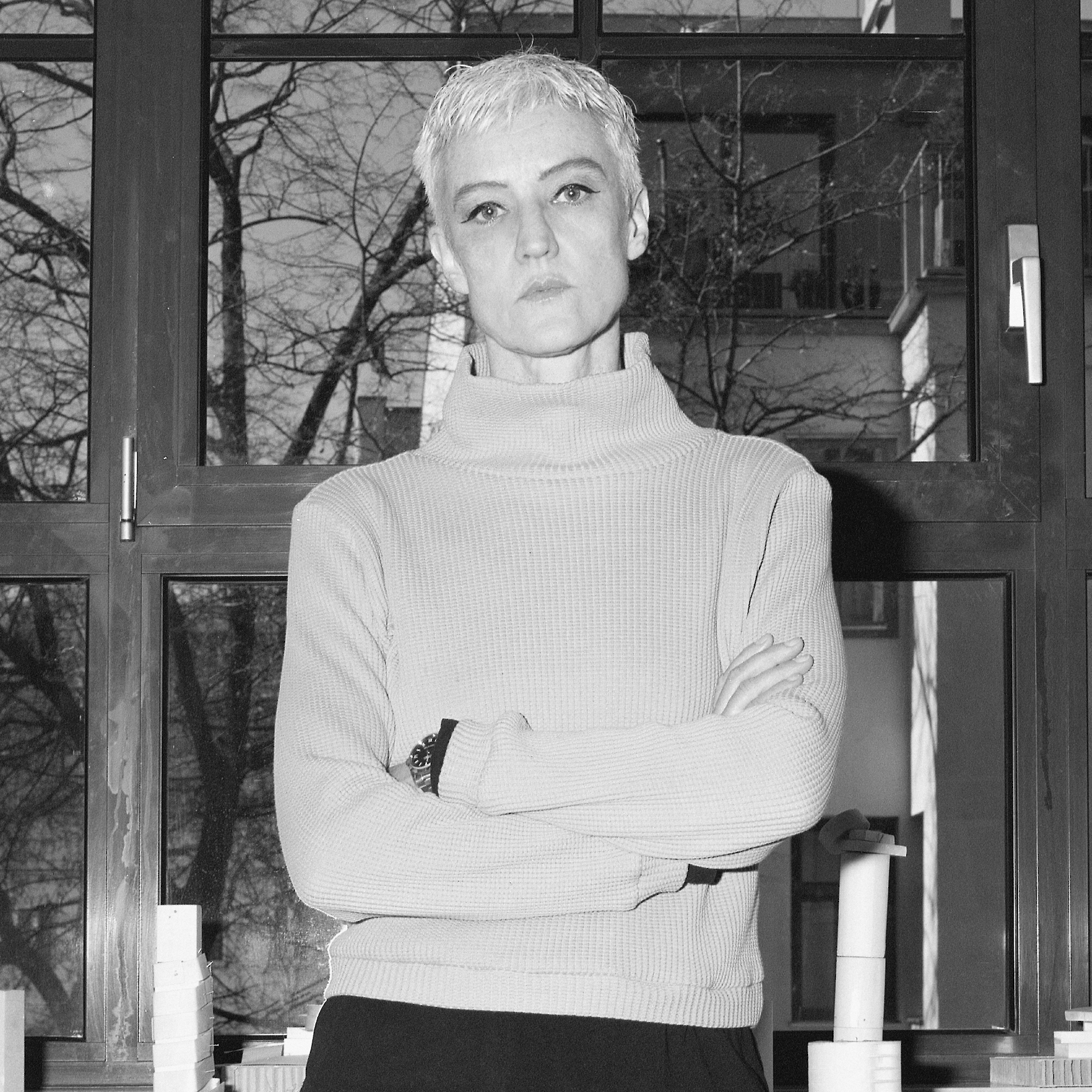
Daniel Bolojan
Senior Architect, Computational Design Specialist
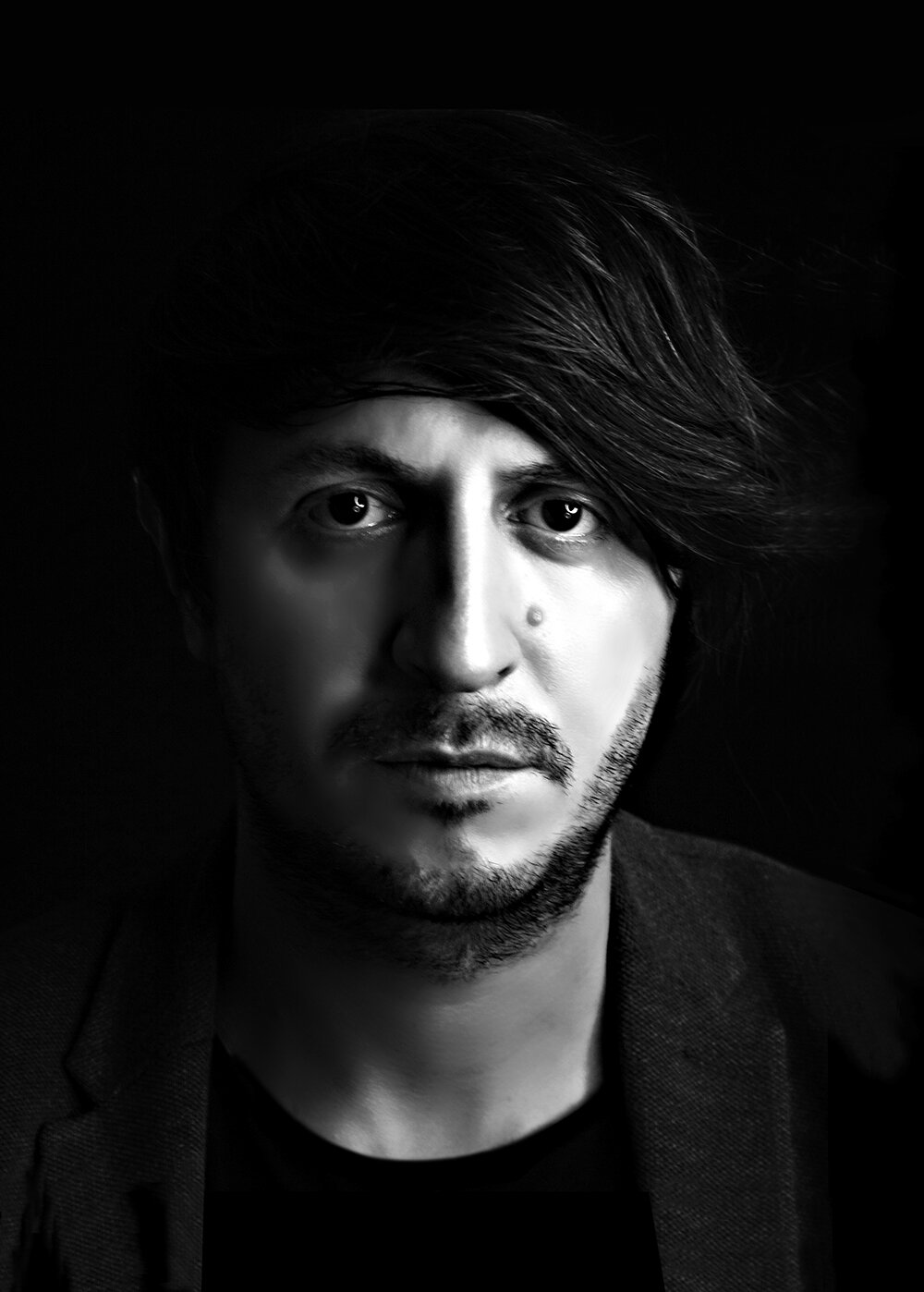
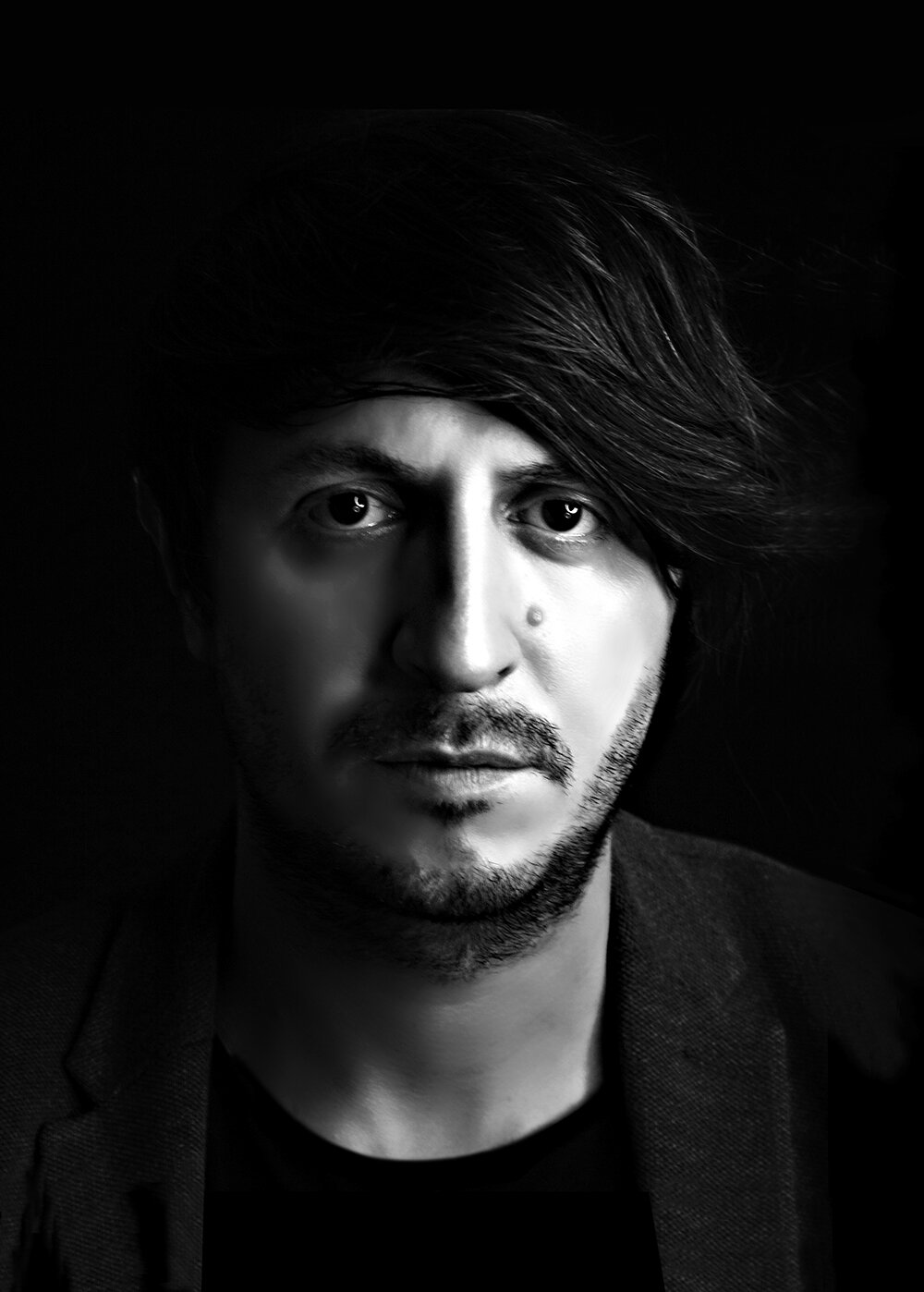
Dina Auni Issa Khozouz
Junior Architect
Index
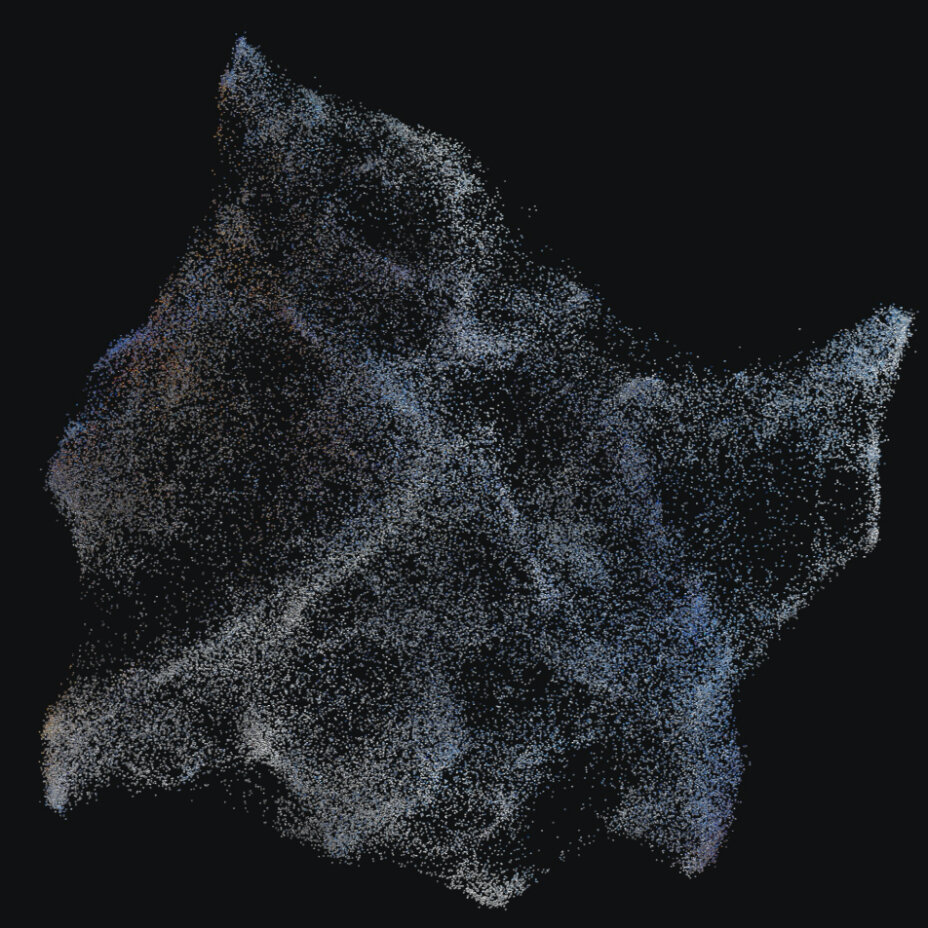
CHBL Universe
Coop Himmelb(l)au
The ability of a machine to execute tasks that are typically associated with intelligent beings.
Deep learning is a class of techniques that are primarily concerned with representation-learning methods. Representation learning can be defined as a collection of methods that allow machines to read raw data and automatically learn data representations.
Explorations that refer to aspects of form.
Explorations that refer to the inner organization of buildings.
Explorations that refer to performative aspects of form and construction assemblies.
The subcategory of machine learning defined by the use of labeled dataset to train a neural network to classify and predict outcomes with accuracy.
The subcategory of machine learning defined by the use of unlabeled dataset to train a neural network.
Learning feature representation of a dataset.
Bounded to the data set.
Not bounded to the data set.
The technique that uses an unpaired dataset to learn the mapping of transferring pictures from one source domain to another.
n-dimensional space containing features, that encodes internal representation of the dataset.
The technique to reduce the high dimensional space into a low dimensional representation while retaining the relations of the original data
What is Deep Himmelblau?
Deep Himmelblau is the result of the cumulative research effort undertaken by Coop Himmelb(l)au which operates at the intersection between architecture, practice, and AI / Deep Learning.
Deep Himmeblau is an experimental research project led by Design Principal Wolf dPrix, Design Partner Karolin Schmidbaur, CHBL's Computational Design Specialist Daniel Bolojan, and Computational Designer Efilena Baseta which explores the potential of teaching machines to interpret, perceive, to be creative, propose new designs of buildings, augment design workflows and augment architect’s / designer’s creativity. Deep Himmelblau is currently the most advanced research dealing with the design potential of AI / Deep Learning undertaken by any architectural office.
What is Deep Himmelblau’s main aim?
There is a very interesting comment about the relationship between the creator / designer and his operating medium / tools -”First we shape our tools, thereafter they shape us”, John Culkin, 1967. Similarly, the research enquires about the future impact of AI on the role of architects / designers and the relationship between new technologies / tools and designers. What role should AI play in the design process? Should the role of AI be to replace architects / designers? Or should it have a design assistant role to interacting with designers / architects to augment design workflows and creativity?
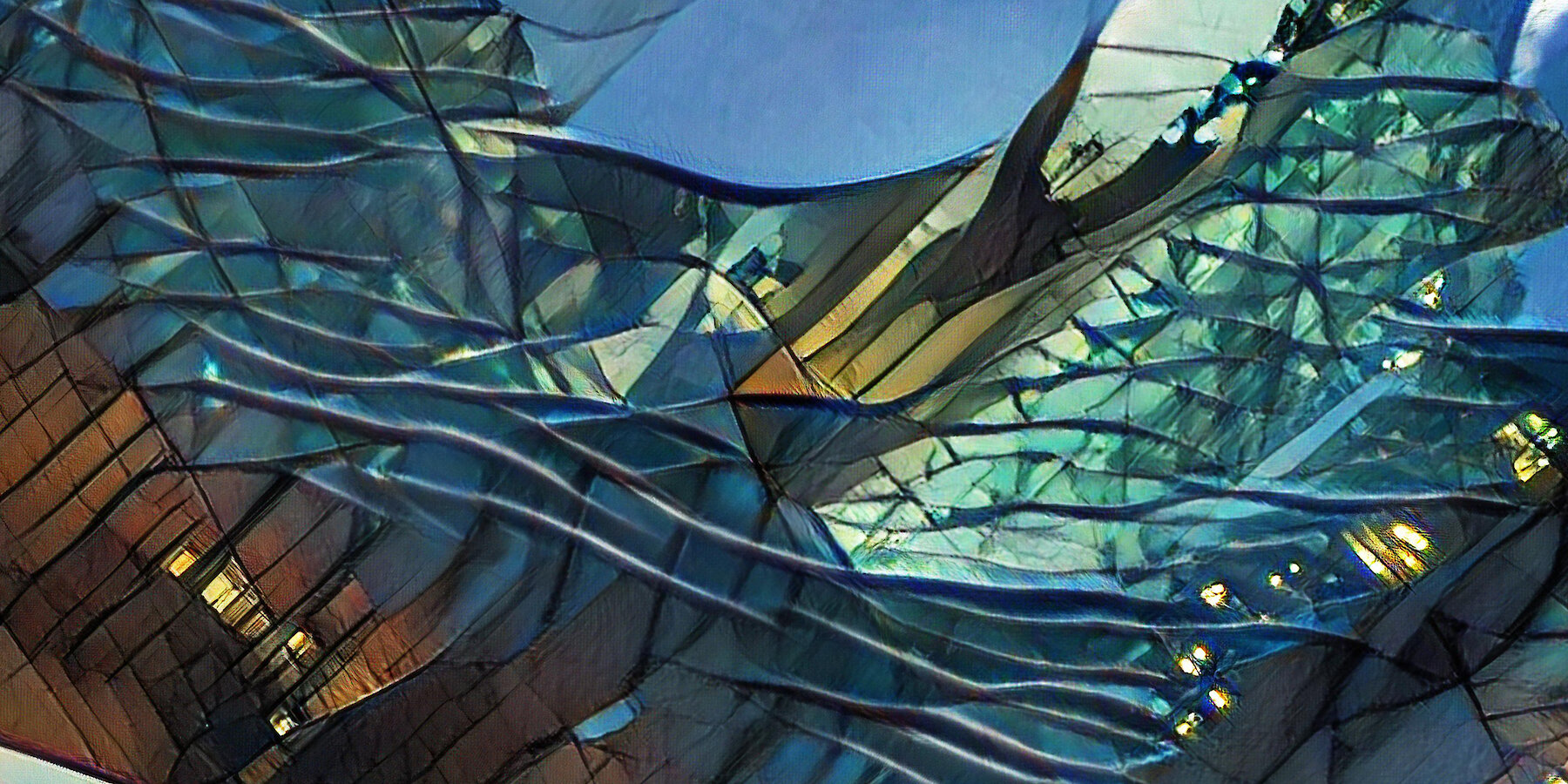
Presentation time laps - DigitalFUTURES
Coop Himmelb(l)au
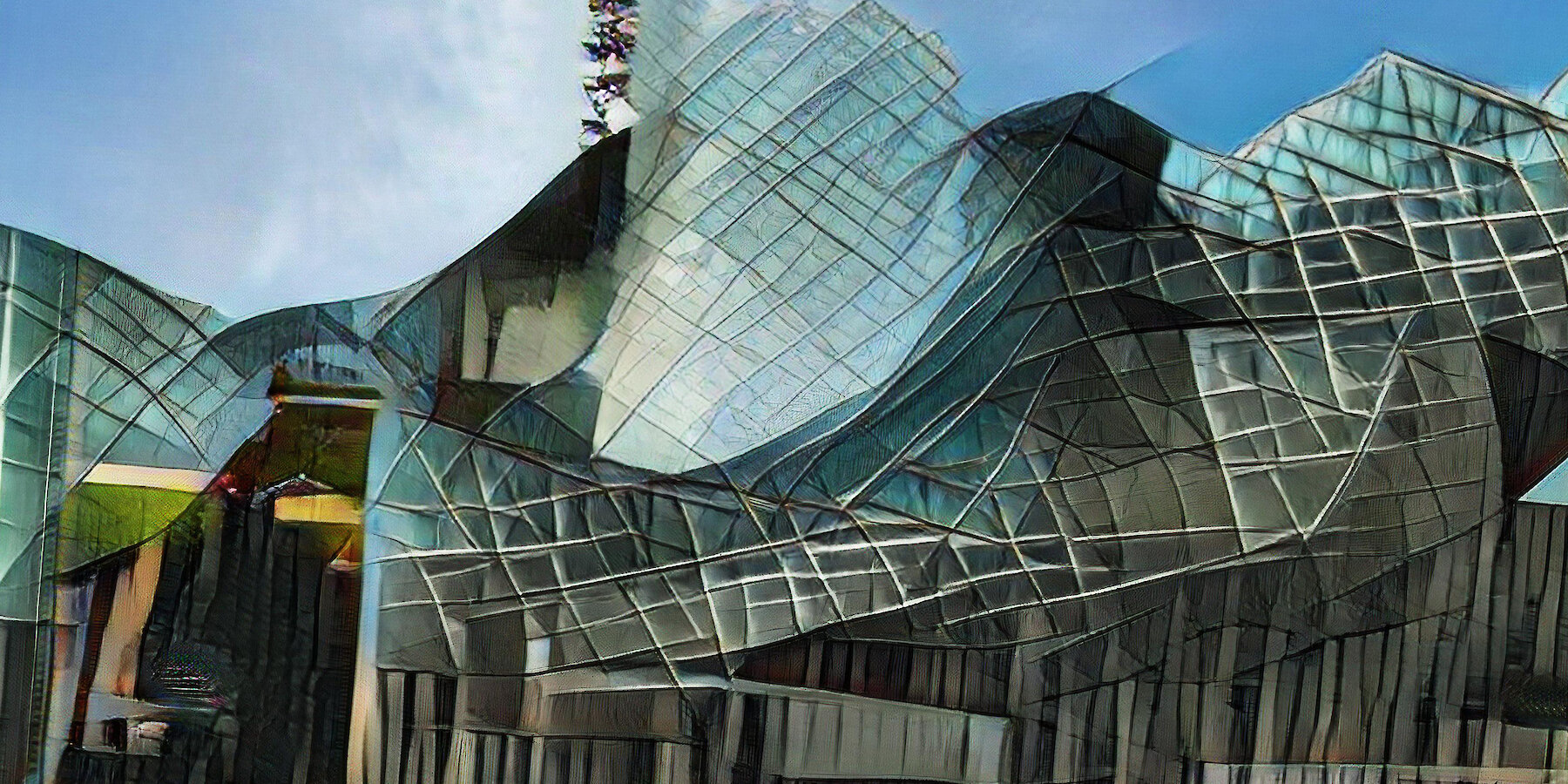
Cloud augmentation
Coop Himmelb(l)au